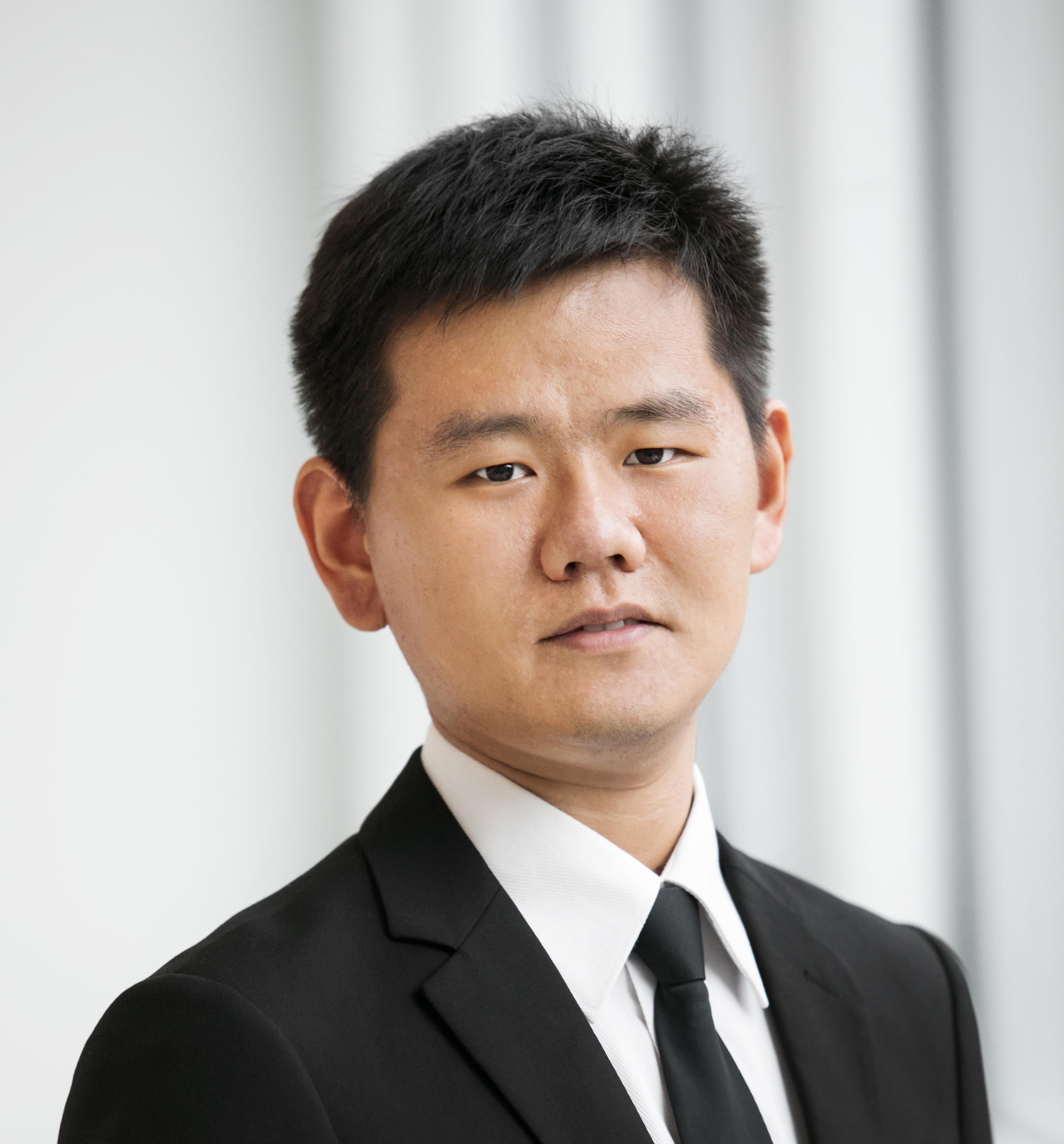
Han Chen
Biography
I am a lecturer in marketing at Monash University. I employ econometrics and causal inference, lab/field experiments, and machine/deep learning to solve marketing problems in the domains of digital advertising, price promotion, product innovation, and human-algorithm interaction. Broadly, my research examines 1) how firms effectively use marketing mix tools to influence consumer behavior in the era of artificial intelligence and the digital world, and 2) how marketers use machine learning algorithms to boost the efficacy of marketing mix tools. My research revolves around (1) the substantive domain of consumers’ responses to marketing messages/disclosures and recommendations, with a special focus on their sequential, dynamic, and unintended effects, and (2) the optimization of firms' sequential marketing interventions.
Education
Ph.D., Marketing
-
Temple University, PA, USA
Dissertation: Marketing Messages, Sequential Effects, and Optimal Disclosure
Master, Management Science and Engineering (ranked 1/31 in class), 2017
-
Northeastern University, China
Thesis: Measurement and Evaluation of User Interactive Experience and Product Design Implications: A Multi-Method Approach Applied to Mobile Shopping Apps
Bachelor, Industrial Engineering (ranked 3/62 in class), 2014
-
Northeastern University, China
Thesis: Optimal Logistics Center Location: Iterative Center-Of-Gravity Method and Application
Research
Ongoing Research
1
Han Chen, Joydeep Srivastava
- Working Paper
2
Han Chen, Yongjun Li
- Work in Progress
Contact
Department of Marketing | 📧: han.chen@monash.edu |
Monash Business School, Monash University | 🌐: hanchenresearch.github.io |